Industries at large have significantly benefited from the development of new technologies, one such technology being artificial intelligence, or AI. AI is used to automate tasks, improve efficiency, and make better decisions, and is already present in our lives in many ways, from the self-driving cars we see on the road to the virtual assistants we use to control our smart home devices.
AI-assisted maintenance is a solution that refers to the integration of AI technologies into the maintenance processes of industrial equipment, machinery, and systems. It is a new category of maintenance, joining current practices such as reactive, preventive, predictive and prescriptive.
These current maintenance practices have existed on the market for a long time. However as technology progresses, maintenance needs have also evolved. The increasing complexity of equipment, the rising cost of downtime, and availability of data and data collection devices have encouraged the growth of systems that incorporate innovative technologies. The assisted maintenance category is the combination of prescriptive and predictive techniques, positioning itself at the forefront of risk management, and optimizing asset performance.
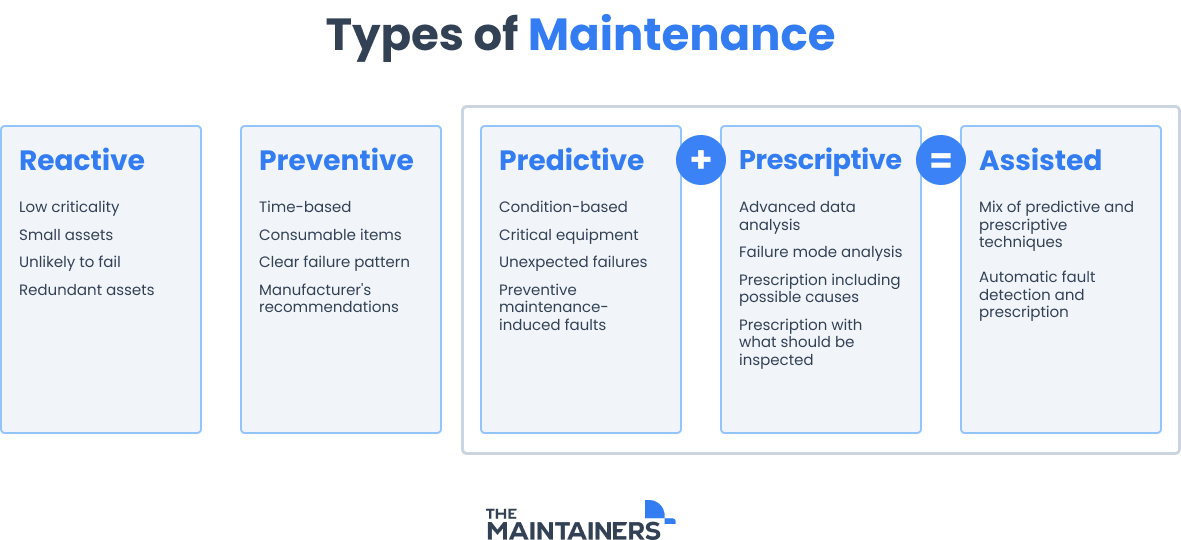
The following subcategories are found within this category:
- AI Assisted Maintenance;
- Augmented Reality (AR) Assisted Maintenance;
- Virtual Reality (VR) Assisted Maintenance;
- Maintenance Assisted by Industrial Internet of Things (IIoT);
- CMMS/EAM Assisted Maintenance;
- Maintenance Assisted by Additive Manufacturing (EM);
- 3D Technology Assisted Maintenance
How Does it Work?
This AI-based approach is mostly driven by the continuous collection of data through online condition monitoring, which makes it possible to keep track of machine performance in real time.
Sensors, IoT devices, and other data sources continuously collect information about various parameters of the equipment, such as temperature, vibration, pressure, current, and more. This data is sent to a centralized system for analysis, often managed by an EAM, a platform that centralizes information on maintenance, intervention history and scheduling.
Relevant features or characteristics are extracted from the raw data, providing insights into the behavior and health of the equipment. Feature extraction can involve techniques such as signal processing and statistical analysis.
Machine learning algorithms, including supervised and unsupervised methods, are trained using historical data. These algorithms learn patterns, correlations, and anomalies from both training and collected data, enabling them to make predictions and decisions. The data extracted from assets are then compared with these learned patterns and algorithms.
If the current data deviates significantly from expected behavior, the system identifies it as an anomaly or potential issue, whether it be equipment malfunction, wear and degradation, or even imminent failure. This enables maintenance teams to address issues before they lead to failures, or take immediate action when required.
Implementing AI to a maintenance routine also builds schedule optimization. Based on the collected data, schedules can be based on equipment condition rather than fixed time intervals, reducing unnecessary checks and maximizing asset uptime. This also means root causes of failure are more likely to be identified, as well as more efficient resource allocation to critical assets or components with higher probability of failure.
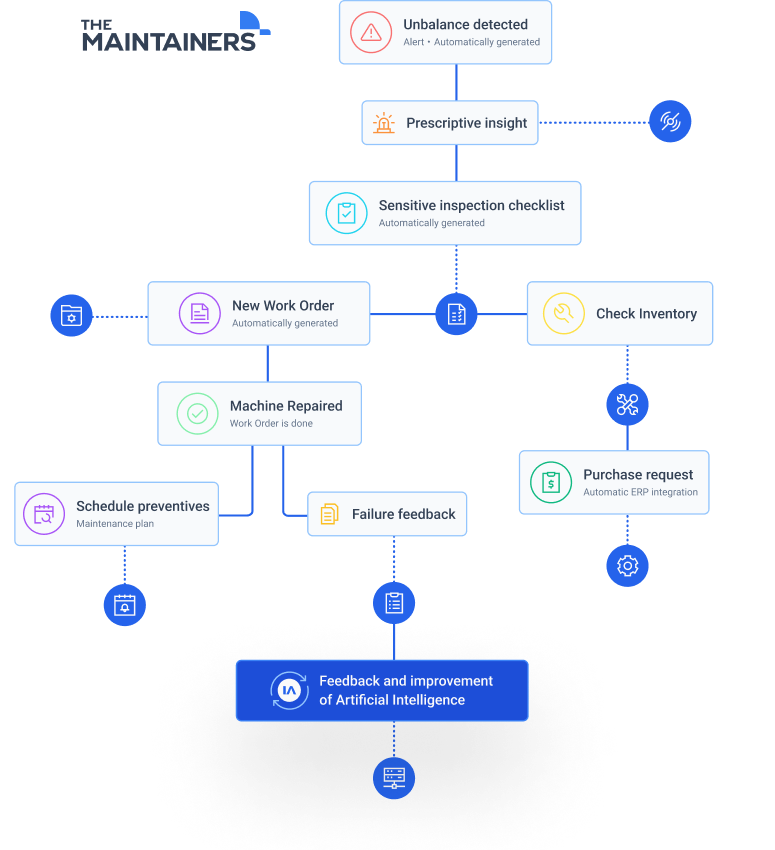
Even with all the analytical capacity of Artificial Intelligence, human action is essential to provide data and analysis that complement the work of AI, also known as human-in-the-loop feedback. While AI technologies can provide predictions, recommendations, and insights, human assertiveness ensures that experienced professionals oversee and validate the actions taken based on the system’s output.
Like any other tool, AI provides maintainers at all levels with the potential to do a job faster, better, and more efficiently, and the key lies in knowing how to use it effectively. Workers that utilize AI understand their assets better, are two steps ahead of potential issues, and are at an advantage over workers that don’t advance their knowledge of new technology!
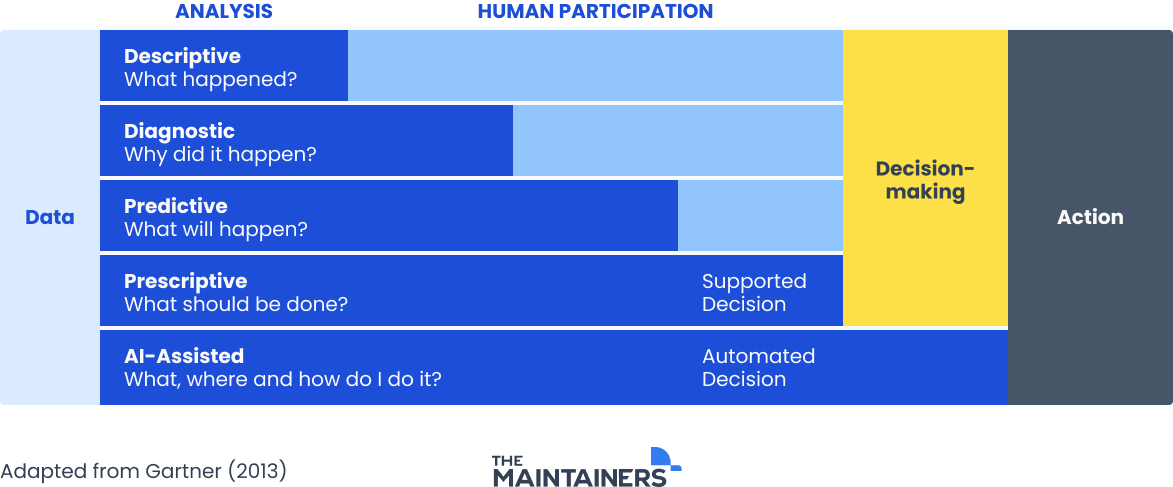
Successful AI-assisted systems offer technical courses of action built off the machine data, manufacturer manuals and feedback from online condition-monitoring sensors, however it is ultimately up to a human’s participation to assess several factors before moving forward with a solution.
Here’s how human assertiveness plays a role in AI-assisted maintenance:
- Interpretation of Results: Human experts interpret the predictions and recommendations provided by AI algorithms. They assess the significance of anomalies, predicted failures, or maintenance alerts based on their domain knowledge and experience.
- Contextual Understanding: Human operators bring contextual understanding to the decision-making process. They consider factors that might not be captured by AI algorithms alone, such as operational priorities, business objectives, and real-world constraints.
- Critical Judgment: In situations where AI predictions are uncertain or conflicting, human experts exercise critical judgment to make informed decisions. They weigh different sources of information and determine the most appropriate course of action.
- Risk Assessment: Human assertiveness involves evaluating the risks associated with implementing AI-recommended maintenance actions. This includes considering the potential consequences of acting on AI insights versus deferring or modifying maintenance activities.
- Complex Problem Solving: Maintenance scenarios involving complex systems or unique situations may require creative problem-solving that draws on the expertise of human operators. AI algorithms might not have encountered similar situations in their training data.
- Adapting to Context: Human assertiveness allows for adaptability in dynamic situations where the AI model’s predictions might not align perfectly with real-time conditions. Human experts can adjust strategies based on real-world changes.
- Accountability: Human experts are accountable for the decisions made, especially in critical scenarios. They take responsibility for actions taken based on AI insights.
- Continuous Learning: Human experts contribute to the continuous improvement of AI models by providing feedback on predictions and outcomes. This helps refine the models and improve their accuracy over time.
- Ethical Considerations: Human assertiveness ensures that ethical considerations are taken into account when making decisions based on AI insights. This includes avoiding biased decisions and ensuring that human values are upheld.
- Learning from AI: Human experts can learn from AI-assisted recommendations and predictions. This exchange of knowledge between human operators and AI systems creates a feedback loop that enhances both human expertise and AI capabilities.
AI-assisted Maintenance Applications
Assisted maintenance is now a reality in industries and manufacturing facilities worldwide. In the aerospace sector, for example, advanced floorplan systems monitor equipment condition in real time, providing a comprehensive view of the status of each piece of equipment on the production line.
If there are any problems or anomalies, the system immediately identifies and notifies operators through illuminated indicators. Faulty equipment is signaled with either red or yellow lights, depending on the severity of the issue. With data easily accessible on mobile phones, remote access to collected information gives maintenance experts greater flexibility and mobility. This gives them greater flexibility and mobility, so they can quickly assess equipment conditions, identify faults, and investigate the root causes of problems.
AI can also generate detailed reports that include spectral analysis, which helps identify the failure modes responsible for increased vibrations in particular equipment, such as unbalances, lubrication problems, or bearing wear. This information can then be used to estimate the remaining useful life of the component or asset.
For example, if a centrifugal water recirculation pump has high vibration levels in all directions, AI can use spectral analysis to identify high-frequency random noise. This is a distinct indicator of cavitation, a well-known phenomenon in this context. AI can then generate a prescriptive report that details the failure mode, severity, possible causes, and what needs to be inspected.
Based on these results, the maintenance management system (CMMS/EAM) can automatically generate work orders containing all the necessary information for execution: specific procedures for each failure, materials, tools, documents, and manuals. This allows managers and supervisors to quickly direct their teams to the required activities and facilitate collaborative troubleshooting to resolve the issue. Work orders are integral to a successful maintenance management program.
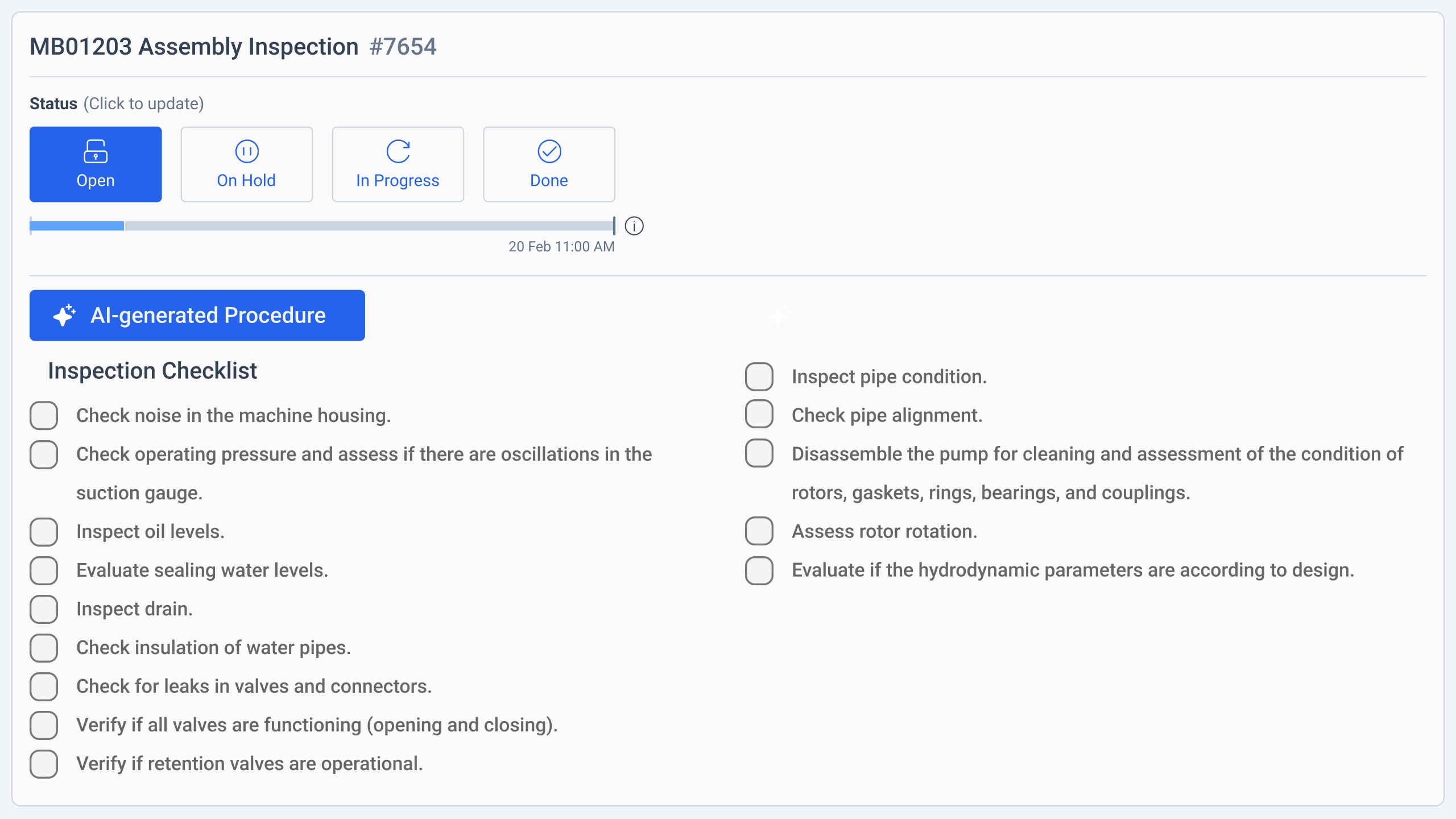
In the case of the centrifugal water recirculation pump, AI might also identify changes in the pump suction pressure. This could indicate that the underlying cause of the cavitation issue is not the pump itself, but rather a different stage in the process.
With the work order already created by the maintenance team, technicians can be directed to investigate the earlier stages of the process, rather than focusing solely on the pump. This strategic shift can lead to improved productivity and faster execution of inspections and repairs, highlighting the agility and efficiency gained through this approach.
Another example of AI’s capabilities is its ability to recommend future actions. For example, AI can be used to determine the optimal time to replace an asset when maintenance costs exceed the asset’s total replacement value. By constantly monitoring these metrics, AI can identify the right time to trigger an alert, signifying that it is time to replace the asset.
AI’s continuous analysis of real-time data creates a virtuous cycle of learning and improvement. Each new diagnosis and prognosis provides the system with valuable information that helps it refine its ability to identify potential failures. This makes the system more assertive in its predictions, which leads to even more accurate diagnoses and prognoses—increasing accuracy and reliability over time.
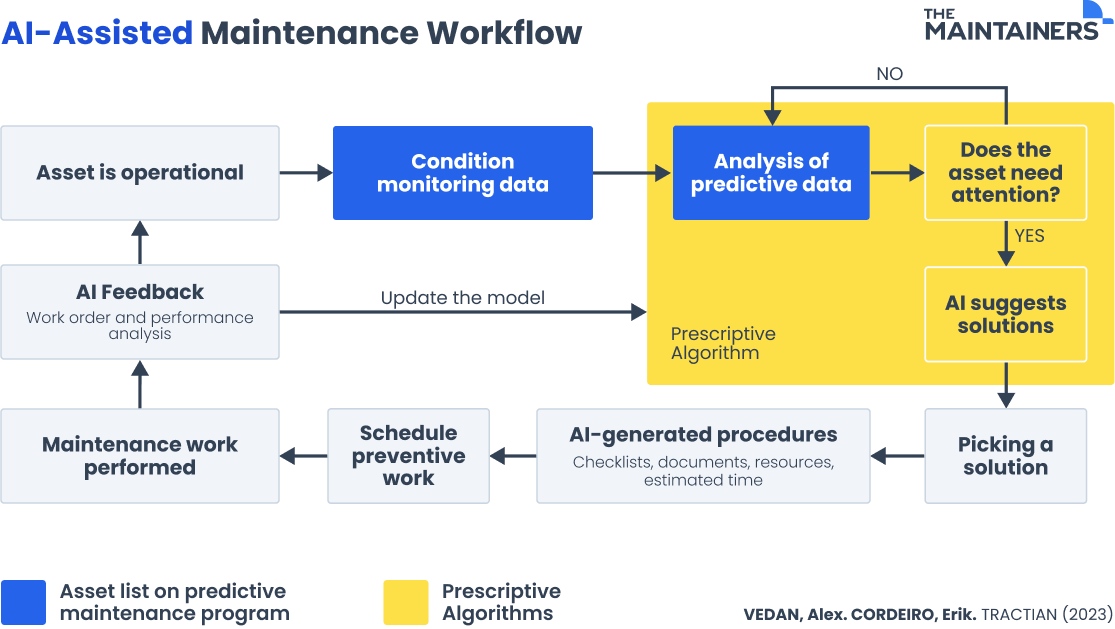
AI-assisted maintenance represents a revolutionary shift in the way we approach industrial maintenance. The ability to predict and prevent problems before they happen, combined with lower operational costs, makes this approach a unique competitive advantage across different industries. By combining cutting-edge technology with expert technical knowledge, systems can deliver precise diagnoses, reliable prognoses, and effective preventive measures, ensuring operational excellence and consistent reliability across industries.
Assisted maintenance is a powerful tool that can optimize operations and reduce costs, and reliably improve the efficiency, productivity, and safety of industrial operations. The implementation of AI is propelling industries towards a future of enhanced performance and continuous innovation.